THANK YOU FOR SUBSCRIBING

Applying Artificial Intelligence Technologies in your Organization
Sophia Van, Global Health Technology Strategy Leader, Mercer Marsh Benefits

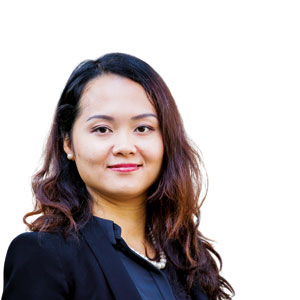
Sophia Van, Global Health Technology Strategy Leader, Mercer Marsh Benefits
According to Gartner Hype Cycle 2017, artificial intelligence (AI) is approaching the peak of its hype. We are seeing mass awareness of its potential to be the most disruptive class of technologies in the next 10 years due to exponential growth of data and on-demand computational power. Organizations are eagerly wishing to dip their toes in the waters of experimentation (85 percent of CIOs will be piloting AI projects by 2020), but many do not know how to begin. The excessive use of AI as a general marketing term has led to much confusion and imprecise thinking. The misunderstanding starts with what AI, actually encompasses (machine learning, deep learning, natural language processing, etc.) and its difference and relation with complementary technologies (robotic process automation, etc.). For example, many startups make exaggerated claims about AI adoption, whereas in fact they are actually using rule-based automation. Many IT vendors’ sales pitches focus too much on the elements of AI that are still in the development stage and that have minimal immediate practical business application.
However, despite the noise, AI is not all hype. Task-oriented AI has proven effective at solving specific business problems, delivering tremendous value. Organizations need not wait for the mainstream adoption of AI, which will take a few more years, but can start the race with effective strategies today.
AI in the Insurance Industry
To succeed with AI, organizations need to cut through the hype and pick the low-hanging fruit. AI is not one single super-intelligent robot that can think and act like humans. It is in fact a vast spectrum of diverse technologies, so organizations need to be very clear on what business issues they want to solve (that is, establish usage cases) and what specific technologies can help.
Because data are both the foundation of AI and the lifeblood of the insurance industry, AI is naturally expected to have a profound impact on this industry. Certain levels of AI have already been adopted within the industry, and below we outline the four main applications.
• Automation
AI has been applied in the insurance industry in the form of automation of tedious processes for which many unstructured data need to be analyzed and there is no value in having a human doing it.
Claims processing is one area that has been successfully AI-augmented. Usually, training algorithms are used to extract information from unstructured and semi-structured data sources (incoming claims, correspondence, receipts, underwriter reports, checks and complaints, etc.) and transpose that data into the right document stores and queues ready for processing by handlers and systems. Such an AI system can review documents in seconds with high accuracy, all while continually learning. Insurers that have applied AI to their claims processes are realizing increased productivity and service quality.
The insurance industry is driven by lots of data, and many of its operations are repetitive and still very manual, so AI is a clear benefit to insurers in automating processes and redeploying humans to use their brains for more creative and innovative tasks. It is expected that generically eighty percent of these types of tasks will be done by machines in the near future. At Mercer, we’re deploying and scaling up AI process automation for repetitive and process-oriented deterministic tasks.
• Chatbots
Chatbots are clearly on the rise as many insurance players embrace the technology to grow skills for wider adoption. According to the technology firm Juniper Network, chatbots will enable up from $20 million in cost savings in 2017 to over $8 billion in savings per annum by 2020 globally. By 2022, banking and healthcare services utilizing bots can expect average timesavings of just over four minutes per enquiry, equivalent to $0.50 to $0.70 in savings per interaction.
Four key steps to enabling the success of a bot project include defining the right use case, designing the conversation flows, choosing the most appropriate tech stack and developing an agile methodology to improve bot performance based on collected analytics before scaling up. At Mercer, we have successfully piloted several chatbots, one of which was in a finalist in the Singapore Fintech Awards in 2017, and we are scaling up our chatbots initiatives globally.
Task-oriented AI has proven effective at solving specific business problems, delivering tremendous value
• Algorithmic Decisions or Advisory
This group of applications includes scoring and risk-modelling, recommendation engines, virtual underwriter assistants, virtual agents and brokers, robo-advisors, and algorithmic models to extract knowledge and predictions from large and diverse data sets to mimic human intelligence in decision-making. With the ability to analyze “big data” (that is, analyzing very large volumes of data with high velocity, variety and veracity), AI can accomplish years of human work in moments. Therefore, with AI, personalized solutions for “segments of one” from millions of data points around customer profiles and behaviors are possible. AI-powered recommendation engines, or virtual agents, are able to understand customers’ needs at a much more in-depth level and can match them with the best insurance products. This capability is crucial in the insurance digital ecosystem model where insurance players try to curate solutions from ecosystem partners to provide users (many) choices. Such intelligence helps consolidate personalized choices. AI-powered recommendation engines and robo-advisors are also important for the “insurance on demand” model, as their intelligence helps insurance platforms deliver customized coverage for specific items and events.
Another area in which AI is assisting human decision-making is underwriting. AI prowess can create a powerful new paradigm for underwriting, creating competitive advantage for insurance players—especially important in the current environment of risk homogenization, margin pressures, and price-sensitive markets. AI is facilitating three distinct innovations in underwriting: predictive and dynamic underwriting, evidence-based underwriting, and automated underwriting, allowing more accurate and personalized underwriting using (almost) real-time information at a much faster speed. Searching medical databases globally or collecting and analyzing other evidence, such as electronic medical data from third-party providers can be automated and supported by the combination of many AI technologies (machine learning, natural language processing, etc.). AI models can learn and predict risks from large sets of data, including non-traditional underwriting dimensions such as consumer behavior collected in real-time from internet-of-things devices(for example, driving behavior captured through car telematic devices or health and wellness data from wearables), making highly dynamic, context- or usage-based pricing possible. Cognitive computing will push automated underwriting to a new frontier. Automation of AI predictive risk-scoring algorithms can therefore potentially help solve one of the traditional challenges of the insurance industry: the long, invasive, and complicated underwriting process. However, such intelligence is based on probabilities and past human decisions, which Organizations should not use such algorithmic decisions as a “black-box model,” but rather they need to have the capabilities to understand and scrutinize all predictions and decisions that the machine makes. Overreliance on the AI models that organizations do not fully understand could have significant consequences; companies should employ AI risk assessment and scoring systems as an advisory tool for human oversight.
• Further Applications of AI
The insurance industry is also using AI for fraud detection, image detection, and so on. Many forward-looking players are exploring AI applications across the value chain and operation stages, from product optimization, customer engagement, pricing and underwriting to claims and payment processes. While waiting for business stakeholders to identify use cases and approve budgets, IT departments can start experimenting and upskilling by applying AI to IT processes that are often intensive and sometimes too complicated for humans to do alone.Three Fundamental Issues for Organizations to Consider Before Adopting AI
Three specific issues organizations need to think strategically about from the start of their AI journeys are:
• Creating a sufficient supply of AI skills • Gaining better access to data • Creating a pipeline of use casesBecause AI is a spectrum of technologies, organizations first need to know what problems they wish to solve with what technologies before acquiring and building the corresponding skills. AI feeds on data, and one key inhibitor to the further expansion of AI is the separation of data in silos and having limited access to different data sources. Organizations must think of data as an important class of assets and develop strategies to access, acquire, protect and capitalize on this asset. Last but not least, building trust in AI is an important overarching strategy that covers governance to improve trust across steps from data quality, to data-sharing mechanisms, to data protection, to model accuracy.
Weekly Brief
I agree We use cookies on this website to enhance your user experience. By clicking any link on this page you are giving your consent for us to set cookies. More info
Read Also
Redefining Risk Management: Strategies for a Safer Future
New Hr Capabilities To Face Evolving Technologies
Strengthening The Compliance Fortress In The Banking Sector
Navigating Legal Challenges By Adapting To Technological Shifts
Compliance In The Medtech Industry
How Can The American Trade Finance Companies Manage Present (And Future?) Chinese Mineral Export Control Measures?
Optimizing Customer Experiences Through Data-Driven Strategies
Customer-Oriented And Compliance Mindsets In Claims Management
